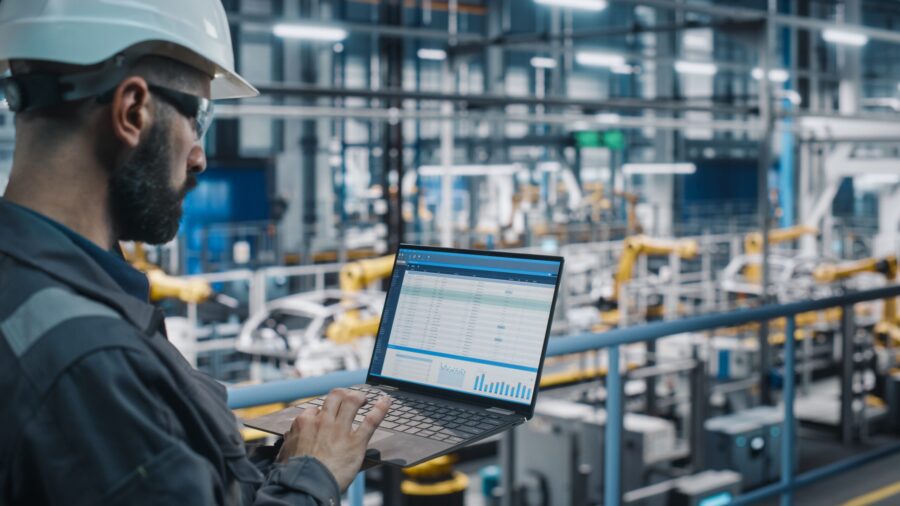
History & Evolution | The Concept of Supply-chain Network, The TOC & the Information Supply-chain | Imagining the future: Supply-chain 5.0 | Supply-chain Analytics Strategy | Roadmap for Building a Data-driven, AI-Powered Supply-chain
Despite the abundance of articles about how Big Data and AI are transforming enterprise supply chains, Gartner’s (consistent) surveys over the past decade have shown that less than half of CDAOs (Chief Data and Analytics Officers) believe their teams have been successful in delivering “some” value (latest Gartner, Mar 2023). In my experience, this is primarily due to organizations making blind investments in advanced analytics platforms and AI tools without first understanding the key principles involved in improving the throughput of the enterprise value chain.
More often than not, the failure lies in CDAO’s strategy, not in the choice of AI tools or technology. A 2020 Gartner survey identified three challenges to supply-chain analytics success:
- Lack of scalable data foundation needed (from the supply-chain)
- Lack of talent and skills relevant to supply-chain (Either you find people who have supply-chain domain experience, or experience in advanced analytics… rare to find people who understand both)
- Lack of clarity on building a supply-chain analytics business case…or, in other words, lack of a coherent supply-chain analytics strategy.
Given this article is rather long, it has been divided into three parts for your ease of reading.
Part-1 of this article examines how data-driven decision-making in supply-chain has evolved over the years, from the earliest rudimentary decision-support systems to the supply chains of the future, which will heavily rely on Bigdata, AI-ML and Generative AI.
Part-2 of this article explains how the enterprise value-chain is not one simple, unitary supply-chain, but rather a complex network, or an interlinked web of multiple interdependent supply-chains that need to work in tandem, and in perfect harmony to ensure the supply-chain performs at 100% & How resolving the bottlenecks in the information-supply-chain is the key to a successful TOC (theory of constraints) implementation for maximizing the enterprise throughput & how applying TOC to Information supply-chain provides a fail-safe methodology for building a data-driven supply-chain.
Part-3 of this article explains the immediate and pressing need for digitizing your supply chain; investing in supply-chain 5.0 is no longer an option, but has become a survival necessity for organizations seeking to remain relevant and competitive in the next decade. It goes on to describe the key features of the digital supply chain, and a broad roadmap for building a Data-driven, AI-powered supply-chain
Part 1: Data-driven supply chain – History & evolution
Is the concept of data driving decisions new?
The concept of “data supporting decisions” is not new. Business intelligence (BI) has been around since the 1960s. However, the amount and availability of data is now far greater than ever, and businesses are relying on data-driven decision-making more heavily than ever before. For those in doubt, I recommend an excellent article from the Journal of Knowledge Economy that chronicles the evolution of the ‘Information-driven decision-making process’ through 1950–2020 (Parra. X & Ors, J Knowl Econ, 2022). Those of us who went to college in the 90s (when Peter Drucker was still alive) may recall being taught that “a manager’s job is to make rational decisions based on whatever data is available at one’s disposal”. Drucker was supposed to have pioneered the use of decision trees to depict the decision-making process (DMP) (Drucker, 1967). Some of the computational models now being extensively used in Artificial Intelligence, like Expert Systems, Neural Networks etc. were actually proposed in the ’70s, but real progress was made in the ’80s and 90’s as computers became more powerful and more affordable. As more and more data became available to support decision-making in the 1990s, decision-support systems were developed (DSS).
Among the most popular applications for DSS in the manufacturing industry were in logistics and supply-chain management.
Decision Support Systems (DSS) for supply-chain: A brief history
A quarter century ago, I used to be a fairly busy SAP consultant, and after several successful full-cycle implementations, I moved companies and switched over to supply-chain consulting…. mostly because I wanted to do something different. Besides, I was not exactly new to supply-chain management; as I had substantial domain experience in Logistics and Distribution-Packaging.
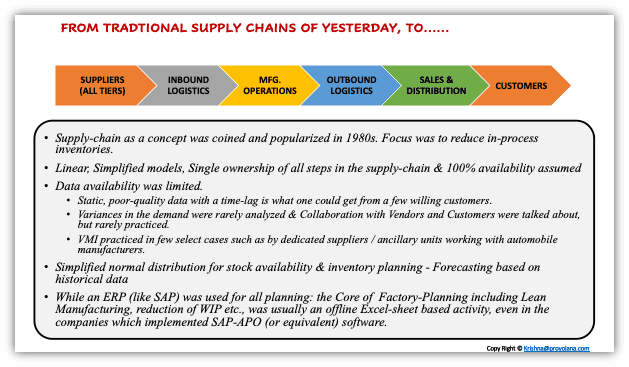
Those days, i2 Technologies dominated the supply-chain and, more specifically, the decision support systems (DSS) space. However, I could notice something was odd about the whole i2 business, right from the day one… ‘i2’ struck me as a poorly thought-out, clumsily designed product; especially when compared to a wondrous and brilliantly designed product like SAP. Most of the i2 product implementations were done by i2 Technologies themselves, and occasionally an odd consultant was brought in from the newly inducted partners. I quickly learned that the i2 consultants charged exorbitant fees, flew only business-class+, …but whenever some customers tried asking pointed questions, they simply threw some jargon at them; while what they said sounded impressive and important, it rarely made sense. Within a few months, I knew enough to deduce there was no real value being delivered to the clients…ironically i2 used to claim they delivered over $ 75 Billion in ’audited savings’ to their customers. Whatever the authenticity of the number, it definitely helped them “sell” their products.
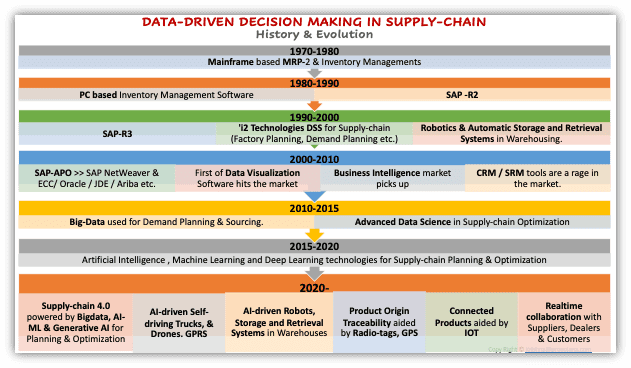
SAP initially worked closely with i2. I remember some of their products were integrated into the SAP and could be configured within SAP. But they eventually fell out for reasons that were never made public, and SAP soon developed and launched APO — a far better-designed product that actually works and delivers value.
In 2002, i2 was fined over $ 1 billion by the SEC (Securities and Exchanges Commission, USA) for misrepresenting its revenues for over five years. Not sure who their external auditor was, and if they also ‘audited’ the savings of $ 75 Billion.
The first decade of the new millennium saw the introduction of a host of new tools and technologies for business intelligence, data warehousing, data visualization, etc., as well as CRM and SRM. Among the best-known are SAP BI-BO, Oracle, Siebel, Ariba, etc. Tableau made its debut in 2003 (it was called Polaris then).
The supply chain software market has seen significant innovation over the past decade with the advent of Bigdata — Advanced Analytics Platforms, AI-ML, IOT — Connected Products, Self-driving trucks, Drones for delivery, etc… Now Generative AI is expected to revolutionize it further.